Conversation Analytics software: The secret sauce for contact center performance
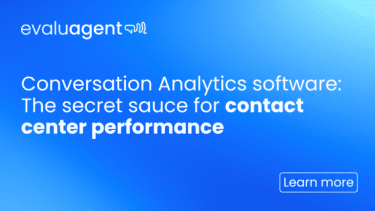
As of Q4 2021, it’s official that 47% of businesses view their contact center as a valuable source of customer insight. One in six contact centers has even achieved the much-coveted status of being considered a “profit center”. So, what’s their secret?
Their superior performance and intelligent operations drive value for teams throughout their organisation – from sales teams, to marketing, to product development. If we could be a fly on the wall of these successful contact centers, we have a niggling suspicion we’d see many of them using Conversation Analytics to unlock actionable insights.
Conversation Analytics uses artificial intelligence, automated speech recognition (ASR), and speech analytics to turn unstructured conversations into text, which can be mined for actionable insight using text analytics and the associated metadata.
In the contact center industry, the technology has been available for well over a decade, but thanks to some sleazy snake oil, is often mis-sold. This leads to a misconception on the technology’s practical capabilities, the role of “AI” and about how Speech and Text Analytics actually works.
In this blog, we explain how it functions and how contact centers are using it to deliver powerful insights and stand-out customer experiences.
In between the interaction and the action, (that is, how you operationalise the insight), Conversation Analytics is carried out in four main stages:
Conversation Intelligence software like evaluagent, connects and centralizes customer interactions and all their relevant metadata – including those from different channels, like voice and text.
Customer interactions over the phone must be turned into text format before they can be analysed. This is done with a transcription engine that can understand the sounds of words and phrases. AI has made this process much faster and more accurate in recent years.
The call transcription results may misunderstand words and phrases that are specific to a particular business such as product names. Certain other words may appear more often than they do in normal speech. The transcription process will usually need to be boosted to ensure these words are correctly identified.
The interaction text is searched to find predefined topics which can be tagged and stored within a searchable database. The content of the interaction is therefore classified into these topics. The keywords and phrases they contain are specified by you or taken from a pre-built theme.
Many contact centers stop here and use the classification process to provide data — and hopefully insight — across their organisation, to drive process change or the prioritisation of which conversations should be passed onto QA teams for a “deeper-dive” evaluation.
Conversations are scored using your pre-defined auto-QA topics, such as process adherence, politeness, or delivery of compliance statements. Automated Quality Assurance Scorecards check against these topics and can score binary or graded outcomes to evaluate the conversation ahead of a QA analyst performing that deeper dive.
Without further ado, let’s take a closer look at how Conversation Analytics can improve your contact center for the good of your customers, your colleagues, and your wallet.
Broadly speaking, Conversation Analytics is used to improve the contact center by identifying high-risk calls that require manual evaluation. Conversation Analytics software doesn’t replace your QA team and their efforts, rather, it helps them use their time more efficiently.
Rather than cherry-picking a handful of contacts for manual evaluation, analytics enables you to filter the conversations that are most important to your contact center. It carries out an initial analysis of up to 100% of conversations to serve as an early-warning system.
This robust and statistically significant analysis not only exceeds the capabilities of your evaluators but allows them to focus their nuanced human analytical skills where they are needed most.
This metric measures the percentage of customer queries that are answered or fixed the first time the customer reaches out to your contact center. It’s not only a measure of efficiency but allows you to make your customer journey much more seamless.
Conversation Analytics can identify and analyse repeat contacts by looking for phrases like “second time I’ve called”, “I spoke to…”, or “called before”. Combining call recording with metadata such as contact disposition (how the agent reported the contact), combined with other data such as first-call resolution and knowledge gaps, can help highlight business issues driving repeat contacts, including knowledge gaps, system issues, disconnected calls, poor service, and customer behaviours.
Analytics gives a broad overview of where inefficient processes are taking place, allowing you to focus on manual evaluation to understand root causes. Depending on what you find, the process for improving FCR might be streamlining communication, better training for advisors, or fixing technology issues.
Average call handle time is a good health check for any contact center, as it provides early warnings for other issues. Dramatic increases across your suite of agents indicate a sudden change or tech problem; consistently high times could highlight overly complex processes; individual agents with long call durations likely need extra training.
The thing about call handle times is that they not only suggest problems – they become a problem. Long call times mean long wait times, feeding back into customer dissatisfaction and agent stress levels to cause rapidly spiralling customer experiences.
Conversation Analytics allows you to stay abreast of changes in call time by viewing trends across agents or call types. Dead air or silence time within the call may alert you to knowledge gaps, whereas call types could help you pinpoint whether it’s arising from a new product or a procedural change. Equipped with root cause analysis, you can decide what remedial action to take, and where and when.
When Conversation Analytics are used to drive insights generation and Quality Assurance, you save significant time identifying and analysing problem interactions. Your quality team works more reliably and can deliver better insights to drive higher standards of agent development.
Demand analysis can identify the need for more useful points of contact for customers, including FAQs and other self-service solutions.
All this, in turn, can reduce demand, increase efficiency, and improve your FCR and average handle time. This allows you to serve more customers and, ultimately, at a lower cost.
While CSAT scores give a digestible snapshot of whether customers are happy with a particular interaction, they leave a lot to be desired when it comes to measuring and improving customer experiences. They are a poor predictor of customer behaviour and only 1 in 26 customers give negative feedback: instead, they just churn.
Conversation Analytics, on the other hand, gives you an unquestionable source of conversation data on customer sentiment, frustrations, and feelings. This allows for a more reliable view of the customer voice than CSAT scores, as well as a more proactive approach to service recovery.
Conversation Analytics taps into the customer voice to identify drivers of customer dissatisfaction so you can quickly roll out targeted action. By looking for words like “angry” and “frustrated”, or key phrases including “beyond a joke” or “can’t be trusted”, it highlights all conversations where customers are unhappy with the product, service, or process delivered to them. This highlights areas to focus on improving the customer experience.
Plus, the insights you gather here don’t just apply to the contact center, but drive value for the organisation at large (skip to the end to read more on this).
Conversation Analytics helps you answer one of the most critical questions in the contact center – why are customers contacting us? It can also section individual contacts into different demand types, and pair conversation insights with metadata to determine when customers are contacting you (and in what volume).
Root cause analysis allows you to identify key trends driving demand, including those that could be avoided by better processes, more customer touchstones, and self-service, or expectation setting. By categorising demand types in terms of value vs irritation, you can identify which ones should be eliminated and which ones you should keep resourcing (and how).
– Mutually valuable: Continue to resource
– Valuable to customers, irritating to contact center: Provide self-service options
– Valuable to contact center, irritating to customers: Simplify processes
– Mutually irritating: Eliminate
Modelling demand in this way contributes to cost reduction, improved customer satisfaction, and overall efficiency.
Contact centers are using Conversation Analytics to build sales strategies and ensure that selling is compliant. For example, Conversation Analytics can align with a customer retention program to ensure that agents are not overselling or mis-selling to keep a wavering customer onboard.
By building topics that identify successful conversions, these conversations can be used as case studies or to develop call center coaching training, so that agents are supported in better sales techniques. Contact centers can feed any insights to the sales department to build better scripts, talk-tracks on demos.
Compliance is a costly issue that can affect any contact center, not just those dealing with highly regulated industries. It’s no surprise that more and more centres use Conversation Analytics to monitor up to 100% of contacts and flag high-risk conversations.
These interactions are pinpointed by keywords hinting at escalation, such as “I want to speak to someone higher”, or “ombudsman”. Contact center can also build their own topics around specific regulated processes within their industry.
Customer conversations are the richest source of information on how your customers feel, what they want, and their journey so it makes sense to share your data with the entire organisation. Some departments may even help pay for it too.
A deeper analysis of the customer voice yields a better understanding of their pain points and how they feel about your marketing communications. This gives the marketing department evidence for their decision about the campaigns and tactics that will resonate most.
You now have a bank of data about when, how, and how long it takes to convert customers successfully. Sales departments can use these trends to build detailed customer journeys and discover key blockers to progression. Armed with key insights about cross-sales, upsells, and even failed sales, they can optimise their sales path with real-world results.
Though reviews are the most obvious source of customer feedback for a product, Conversation Analytics offers insights on how customers feel about a product and any specific lines or features that generate problems (or delight!). Not all customers are inclined to leave reviews – especially if their problem was resolved – but the fact that there was a problem is key information for product teams.
Monitoring compliance in a contact center is a lengthy task and comes with heavy responsibilities. Conversation Analytics allows evaluation of 100% of calls, to flag high-risk contacts, identify whether compliance scripts are read correctly, and provide agent training on problem areas. It’s peace of mind for the compliance team and can save significant time otherwise lost to manual, box-ticking exercises.
Without further ado, let’s take a closer look at how conversation analytics can improve your contact center for the good of your customers, your colleagues and your wallet.
Analytics has an impressive brochure of potential use-cases – but let’s remember that these are (mostly) goals and not out-of-the-box capabilities. Just like a flourishing ecosystem doesn’t spring up overnight, contact centers implementing analytics should think carefully about which seeds to sow and how to nourish them.
Pick one case first; then plan, deploy, and tweak.
And no matter where you are in your QA journey, keep in mind that technology helps – but the real secret sauce of high-performance contact centers is the people within them.